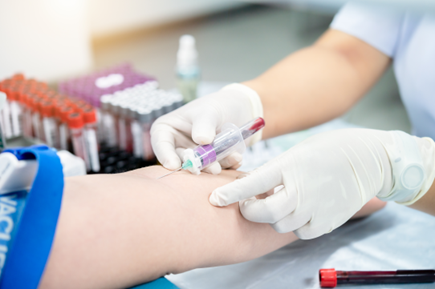
Image source: Adobe stock
MASH (metabolic dysfunction-associated steatohepatitis) is a liver disease, where >5% fat accumulates in the liver along with inflammation and sometimes fibrosis. It is a severe form of MASLD (metabolic dysfunction associated steatotic liver disease) which is caused by just fat deposition in the liver. It is common and affects 25-30% of the global population; out of those 20% progress to MASH. As expected, MASH patients are at higher risk of liver fibrosis, failure, and cancer. The invasive liver biopsy is still a gold standard way of diagnosing MASH. It has various disadvantages, such as poor acceptability, high cost, post-procedure pain, and variation in interpreting the results by pathologists. Thus, there is a critical need for a non-invasive biomarker to diagnose MASH. Many non-invasive diagnostic approaches have been suggested for MASH but they offer moderate accuracy. A recent report by Zhang et al. in Cell Metabolism used 3 biomarkers called N3-MASH to diagnose MASH with 79.7% specificity, 64.4% sensitivity, and 90.7% positive predictive value. They validated it in 3 independent cohorts comprising different ethnicities and locations.
What is the N3-MASH
N3 MASH consists of 3 biomarkers – CXCL10, CK-18, and adjusted BMI. CXCL10 (C-X-C motif chemokine ligand 10) is a key inflammatory mediator that promotes MASH by impairing autophagy. CK-18 (Cytokeratin 18 fragments M30) is a marker for liver cell apoptosis. It is one of the extensively studied biomarkers for MASH but is alone insufficient to use in clinics. Ethnic-specific adjusted body mass index (BMI) was also used in the panel to accurately diagnose MASH.
Study and validation populations
The authors screened 23 markers to diagnose MASLD and MASH – 6 were protein biomarkers and 17 clinical variables. Out of which they shortlisted 7 biomarkers – CXCL10, CK-18, SQLE, HbA1c, ALT, AST, and BMI – using machine learning models. Further to reduce the number of biomarkers, they optimized to 3 biomarkers called N3-MASH – CXCL10, CK-18, and BMI – that gave the best diagnostic value in terms of differentiating between true positives and negative, called AUROC (area under receiver operating characteristic curve). These biomarkers were obtained from the discovery cohort of 184 individuals in Hong Kong comprising 112 MASLD patients with 62 MASH patients through pathological assessment and 72 healthy controls. Its AUROC score was 0.823. The three validation cohorts were from the University of California, San Diego, USA; Wenzhou, China; and Hong Kong. The details of these cohorts are listed below.
Cohorts | Healthy controls | MASLD | MASH in MASLD | AUROC score |
San Deigo, USA | 72 | 112 | 98 | 0.802 |
Wenzhou, China | 0 | 240 | 203 | 0.805 |
Hong Kong | 0 | 103 | 64 | 0.823 |
The 3 biomarkers panel could successfully distinguish MASH patients from MASLD in both discovery and validation cohorts. They showed better diagnostic ability than existing biomarkers – ALT (AUROC- 0.693) and AST (AUROC- 0.714). Furthermore, N3-MASH was also decreased in patients who were undergoing treatment, consistent with the pathological improvement in their liver biopsy with AUROC of 0.857. However, only 48 patients were used for this analysis. Thus, more cohorts should be included to increase the power of the analysis.
Strengths of the study
- It was validated in a diverse population. It was initially tested in the Asian population but then tested in the San Diego, USA cohort, which comprised 47.4% Caucasian, 28.3% Hispanic or Latino, and 17.3% Asian.
- The components of N3-MASH are easy to test and compatible with current testing practices.
- The N3-MASH is specifically designed and optimized for MASH patients to diagnose them and monitor their improvement with treatment.
- It is not influenced by age, gender, ALT, diabetes, or hypertension.
Limitations of the study
- Assessment of liver biopsy varies among the pathologists and represents a small portion of the liver, which may not be a true representation of liver health.
- The CXCL10 and CK-18 measurements need to be optimized once the test becomes commercially available to mitigate potential batch effects and ensure consistent measurements.
Dr. Vinny Negi, Ph.D.
Disclaimer
The editors take care to share authentic information. In case of any discrepancies please write to newsletter@medness.org
The sponsors do not have any influence on the nature or kind of the news/analysis reported in MedNess. The views and opinions expressed in this article are those of the authors and do not necessarily reflect the official policy or position of MedNess. Examples of analysis performed within this article are only examples. They should not be utilized in real-world analytic products as they are based only on very limited and dated open-source information. Assumptions made within the analysis are not reflective of the position of anyone volunteering or working for MedNess. This blog is strictly for news and information. It does not provide medical advice, diagnosis or treatment nor investment suggestions. This content is not intended to be a substitute for professional medical advice, diagnosis, or treatment. Always seek the advice of your physician or another qualified health provider with any questions you may have regarding a medical condition. Never disregard professional medical advice or delay in seeking it because of something you have read on this website.
MedNess is a part of STEMPeers® which is a 501(c)(3) organization registered in PA as PhD Career Support Group. The organization helps create a growing network of STEM scientists that is involved in peer-to-peer mentoring and support.